Uncategorized
The DeepSeek-R1 Effect and Web3-AI
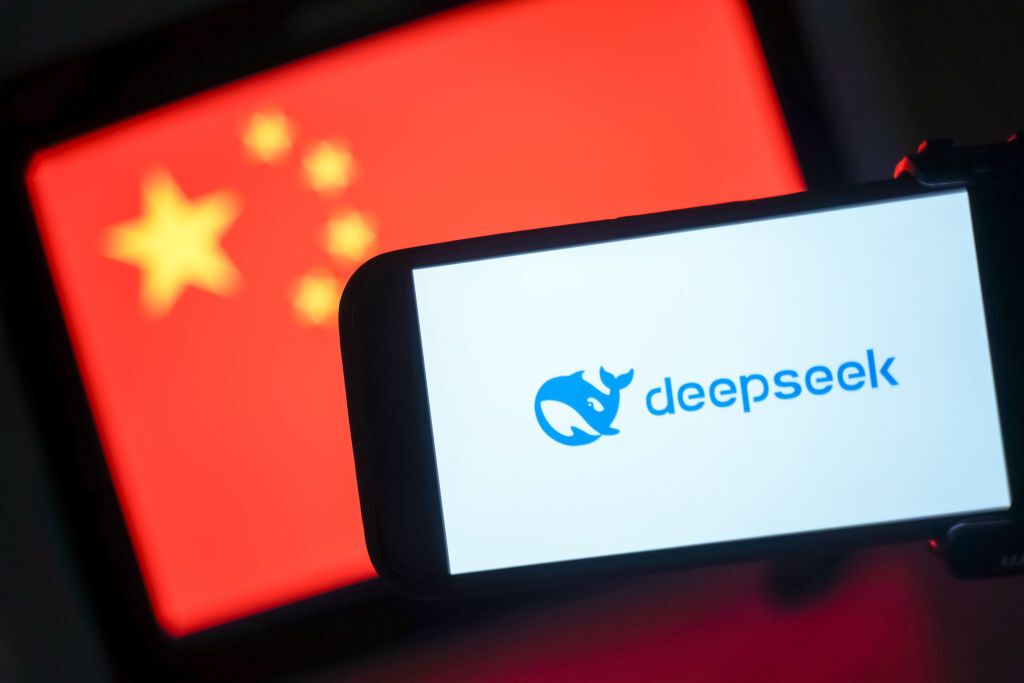
The artificial intelligence (AI) world was taken by storm a few days ago with the release of DeepSeek-R1, an open-source reasoning model that matches the performance of top foundation models while claiming to have been built using a remarkably low training budget and novel post-training techniques. The release of DeepSeek-R1 not only challenged the conventional wisdom surrounding the scaling laws of foundation models – which traditionally favor massive training budgets – but did so in the most active area of research in the field: reasoning.
The open-weights (as opposed to open-source) nature of the release made the model readily accessible to the AI community, leading to a surge of clones within hours. Moreover, DeepSeek-R1 left its mark on the ongoing AI race between China and the United States, reinforcing what has been increasingly evident: Chinese models are of exceptionally high quality and fully capable of driving innovation with original ideas.
Unlike most advancements in generative AI, which seem to widen the gap between Web2 and Web3 in the realm of foundation models, the release of DeepSeek-R1 carries real implications and presents intriguing opportunities for Web3-AI. To assess these, we must first take a closer look at DeepSeek-R1’s key innovations and differentiators.
Inside DeepSeek-R1
DeepSeek-R1 was the result of introducing incremental innovations into a well-established pretraining framework for foundation models. In broad terms, DeepSeek-R1 follows the same training methodology as most high-profile foundation models. This approach consists of three key steps:
Pretraining: The model is initially pretrained to predict the next word using massive amounts of unlabeled data.
Supervised Fine-Tuning (SFT): This step optimizes the model in two critical areas: following instructions and answering questions.
Alignment with Human Preferences: A final fine-tuning phase is conducted to align the model’s responses with human preferences.
Most major foundation models – including those developed by OpenAI, Google, and Anthropic – adhere to this same general process. At a high level, DeepSeek-R1’s training procedure does not appear significantly different. ButHowever, rather than pretraining a base model from scratch, R1 leveraged the base model of its predecessor, DeepSeek-v3-base, which boasts an impressive 617 billion parameters.
In essence, DeepSeek-R1 is the result of applying SFT to DeepSeek-v3-base with a large-scale reasoning dataset. The real innovation lies in the construction of these reasoning datasets, which are notoriously difficult to build.
First Step: DeepSeek-R1-Zero
One of the most important aspects of DeepSeek-R1 is that the process did not produce just a single model but two. Perhaps the most significant innovation of DeepSeek-R1 was the creation of an intermediate model called R1-Zero, which is specialized in reasoning tasks. This model was trained almost entirely using reinforcement learning, with minimal reliance on labeled data.
Reinforcement learning is a technique in which a model is rewarded for generating correct answers, enabling it to generalize knowledge over time.
R1-Zero is quite impressive, as it was able to match GPT-o1 in reasoning tasks. However, the model struggled with more general tasks such as question-answering and readability. That said, the purpose of R1-Zero was never to create a generalist model but rather to demonstrate it is possible to achieve state-of-the-art reasoning capabilities using reinforcement learning alone – even if the model does not perform well in other areas.
Second-Step: DeepSeek-R1
DeepSeek-R1 was designed to be a general-purpose model that excels at reasoning, meaning it needed to outperform R1-Zero. To achieve this, DeepSeek started once again with its v3 model, but this time, it fine-tuned it on a small reasoning dataset.
As mentioned earlier, reasoning datasets are difficult to produce. This is where R1-Zero played a crucial role. The intermediate model was used to generate a synthetic reasoning dataset, which was then used to fine-tune DeepSeek v3. This process resulted in another intermediate reasoning model, which was subsequently put through an extensive reinforcement learning phase using a dataset of 600,000 samples, also generated by R1-Zero. The final outcome of this process was DeepSeek-R1.
While I have omitted several technical details of the R1 pretraining process, here are the two main takeaways:
R1-Zero demonstrated that it is possible to develop sophisticated reasoning capabilities using basic reinforcement learning. Although R1-Zero was not a strong generalist model, it successfully generated the reasoning data necessary for R1.
R1 expanded the traditional pretraining pipeline used by most foundation models by incorporating R1-Zero into the process. Additionally, it leveraged a significant amount of synthetic reasoning data generated by R1-Zero.
As a result, DeepSeek-R1 emerged as a model that matched the reasoning capabilities of GPT-o1 while being built using a simpler and likely significantly cheaper pretraining process.
Everyone agrees that R1 marks an important milestone in the history of generative AI, one that is likely to reshape the way foundation models are developed. When it comes to Web3, it will be interesting to explore how R1 influences the evolving landscape of Web3-AI.
DeepSeek-R1 and Web3-AI
Until now, Web3 has struggled to establish compelling use cases that clearly add value to the creation and utilization of foundation models. To some extent, the traditional workflow for pretraining foundation models appears to be the antithesis of Web3 architectures. However, despite being in its early stages, the release of DeepSeek-R1 has highlighted several opportunities that could naturally align with Web3-AI architectures.
1) Reinforcement Learning Fine-Tuning Networks
R1-Zero demonstrated that it is possible to develop reasoning models using pure reinforcement learning. From a computational standpoint, reinforcement learning is highly parallelizable, making it well-suited for decentralized networks. Imagine a Web3 network where nodes are compensated for fine-tuning a model on reinforcement learning tasks, each applying different strategies. This approach is far more feasible than other pretraining paradigms that require complex GPU topologies and centralized infrastructure.
2) Synthetic Reasoning Dataset Generation
Another key contribution of DeepSeek-R1 was showcasing the importance of synthetically generated reasoning datasets for cognitive tasks. This process is also well-suited for a decentralized network, where nodes execute dataset generation jobs and are compensated as these datasets are used for pretraining or fine-tuning foundation models. Since this data is synthetically generated, the entire network can be fully automated without human intervention, making it an ideal fit for Web3 architectures.
3) Decentralized Inference for Small Distilled Reasoning Models
DeepSeek-R1 is a massive model with 671 billion parameters. However, almost immediately after its release, a wave of distilled reasoning models emerged, ranging from 1.5 to 70 billion parameters. These smaller models are significantly more practical for inference in decentralized networks. For example, a 1.5B–2B distilled R1 model could be embedded in a DeFi protocol or deployed within nodes of a DePIN network. More simply, we are likely to see the rise of cost-effective reasoning inference endpoints powered by decentralized compute networks. Reasoning is one domain where the performance gap between small and large models is narrowing, creating a unique opportunity for Web3 to efficiently leverage these distilled models in decentralized inference settings.
4) Reasoning Data Provenance
One of the defining features of reasoning models is their ability to generate reasoning traces for a given task. DeepSeek-R1 makes these traces available as part of its inference output, reinforcing the importance of provenance and traceability for reasoning tasks. The internet today primarily operates on outputs, with little visibility into the intermediate steps that lead to those results. Web3 presents an opportunity to track and verify each reasoning step, potentially creating a «new internet of reasoning» where transparency and verifiability become the norm.
Web3-AI Has a Chance in the Post-R1 Reasoning Era
The release of DeepSeek-R1 has marked a turning point in the evolution of generative AI. By combining clever innovations with established pretraining paradigms, it has challenged traditional AI workflows and opened a new era in reasoning-focused AI. Unlike many previous foundation models, DeepSeek-R1 introduces elements that bring generative AI closer to Web3.
Key aspects of R1 – synthetic reasoning datasets, more parallelizable training and the growing need for traceability – align naturally with Web3 principles. While Web3-AI has struggled to gain meaningful traction, this new post-R1 reasoning era may present the best opportunity yet for Web3 to play a more significant role in the future of AI.
Uncategorized
Ethereum ‘Roll Back’ Suggestion Has Sparked Criticism. Here’s Why It Won’t Happen
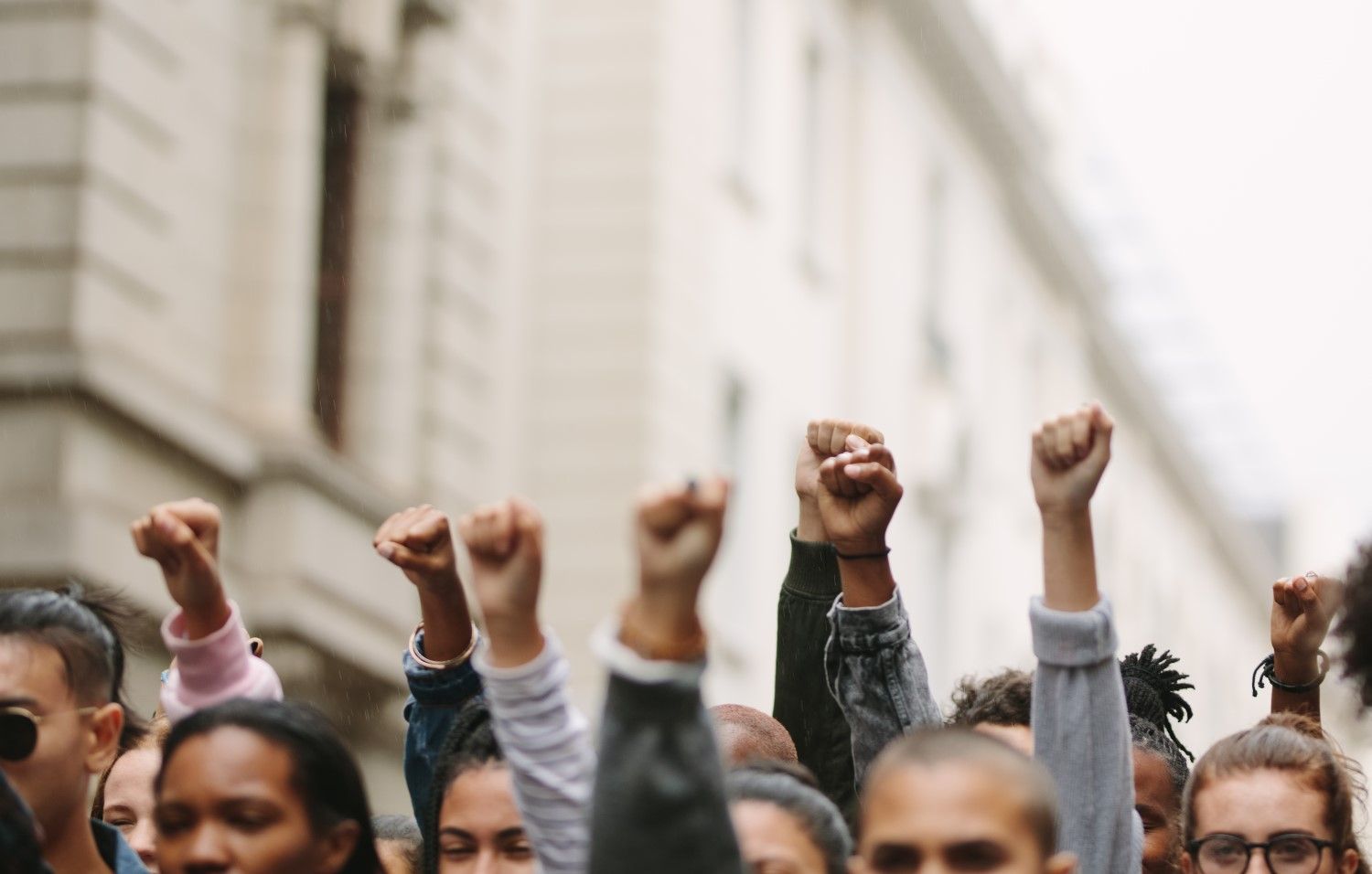
On Friday, cryptocurrency exchange Bybit was allegedly hacked by North Korea’s Lazarus group, which drained nearly $1.4 billion in ether (ETH) from the exchange.
Following the hack, Arthur Hayes, BitMEX co-founder and claiming to be a major ether (ETH) holder, wrote a post on X to Ethereum co-founder Vitalik Buterin on whether he will “advocate to roll back the chain to help @Bybit_Official.” Meanwhile, in an X spaces session, Bybit’s CEO Ben Zhou revealed that his team had also reached out to the Ethereum Foundation to see if it was something the network would consider, noting that such a decision should be based on what the network’s community wants.
Hayes’s post immediately provoked a fierce reaction from the Ethereum community, which was firm in its belief that it wouldn’t happen. Some even questioned whether the BitMEX founder was joking. CoinDesk reached out to Hayes over X to clarify his comments.
Ethereum members, like the core developer teams, are vastly against “rolling back” the network because it would override core elements of decentralization. If Buterin decided on his own that it would happen, then that would be seen as the end of Ethereum’s ethos, which heavily involves various developer teams and other community members when it comes to the health and state of the blockchain.
“Rolling back the chain would give ETH no purpose. What’s the point if you can just change rules,” said user @the_weso in a post on X.
Some outside the Ethereum community pointed to the 2016 DAO hack as an example when $60 million in ETH was stolen. The network went forward with a hard fork, splitting the old network into two, and the new chain continued on as Ethereum.
That hard fork was not a “rollback,” though; it was known as an “irregular state transition.” Ethereum technically can’t “roll back” the network because it relies on an account model, where accounts hold users’ ETH.
At the time of the hack, developers upgraded their nodes to a new client or software. Those who didn’t upgrade their nodes were still on the old chain, which became known as Ethereum Classic.
When the nodes upgraded to the new software, the stolen ETH could move from one Ethereum account address to the next.
“The ‘irregular state change’ that they implemented at the time of the DAO hard fork was this: they airlifted all the ETH in the DAO smart contracts out to a refund contract that would send you 1 ETH for every 100 DAO tokens you sent in,” wrote Laura Shin of Unchained in a post on X.
Uncategorized
Bybit Sees Over $4 Billion ‘Bank Run’ After Crypto’s Biggest Hack
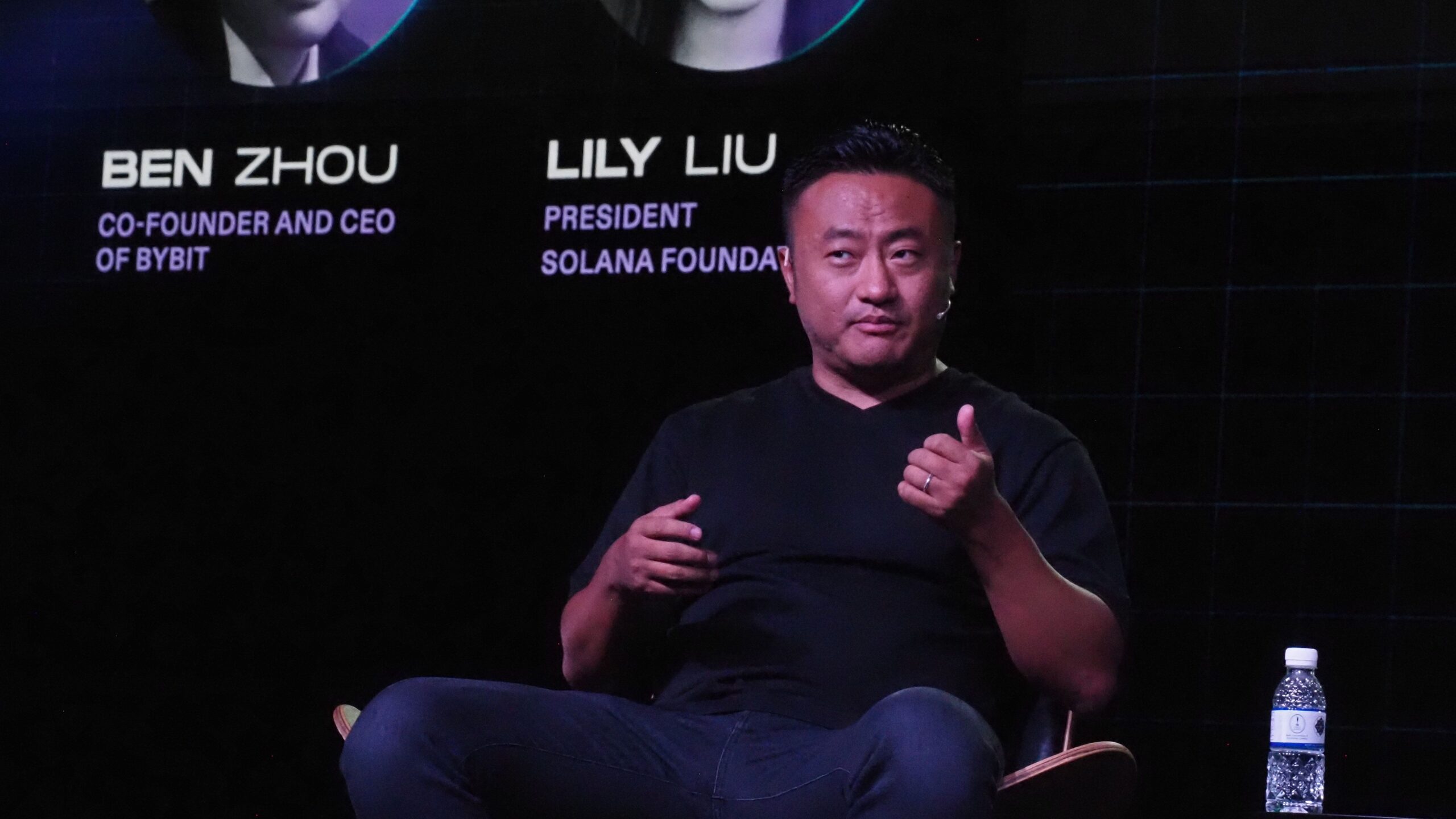
Major cryptocurrency exchange Bybit has seen total outflows of over $5.5 billion after it suffered a near $1.5 billion hack that saw hackers, believed to be from North Korea’s Lazarus Group, drain its ether cold wallet.
The total assets tracked on wallets associated with the exchange plunged from around $16.9 billion to $11.2 billion at the time of writing, according to data from DeFiLlama. The exchange is now looking to understand exactly what happened.
In an X spaces session, Bybit’s CEO Ben Zhou revealed that shortly after the incident, he called for “all hands on deck” to serve their clients with processing withdrawals and responding to inquiries about what was going on.
During the session, Zhou revealed that the security breach saw the hackers make off with roughly 70% of their clients’ ether, which meant that Bybit needed to quickly secure a loan to be able to process withdrawals. Yet, Zhou found that ether wasn’t the most withdrawn token, with most users instead withdrawing stablecoin from Bybit.
The exchange, Zhou noted, has reserves to cover these withdrawals, but the crisis deepened as, in response to the incident, Safe moved to temporarily shut down its smart wallet functionalities to “ensure absolute confidence in our platform’s security.”
Safe is a decentralized custody protocol providing smart contract wallets for digital asset management. Some exchanges integrated Safe, which allows users to maintain custody of their funds and has multisig functionality to enhance the security of their cold wallets.
While the exchange had reserves to back up users’ withdrawals, $3 billion worth of USDT was in a Safe wallet that had just been shut down as the wallet moved to understand the situation, according to Zhou.
On social media, Safe said that while it had «not found evidence that the official Safe frontend was compromised,» it was temporarily shutting down «certain functionalities» out of caution.
While Zhou and Bybit’s team were figuring out how to securely withdraw their $3 billion, withdrawals were mounting. Within two hours of the security breach, the exchange was facing requests to move over $100,000 off its platform, Zhou revealed.
Responding to the situation, Zhou told his security team to engage Safe to “find a better way to get this money out.” The team ended up developing new software with code “based on Etherscan” to verify the signatures “on a very manual level” to move the stablecoins back to their wallet and cover the withdrawal surge.
The exchange’s team had to remain up all night to be able to fulfill withdrawals, according to Zhou. As the exchange managed to move the $3 billion in stablecoin reserves, it was facing a bank run of “about 50%” of all the funds within the exchange.
Zhou said that since the incident, the exchange has moved a significant amount of funds off of Safe cold wallets and is now determining what system it will use to replace Safe.
Pushing to «Roll Back» Ethereum Was not Off the Table
Since the security breach, Bybit has engaged authorities. During the session, Zhou said that the Singaporean authorities took the issue “very seriously” and that he believes it has already been escalated with Interpol.
Blockchain analysis firms, including Chainalysis, were engaged. Zhou said, “As long as Bybit is there and continues to track [the stolen ether], I hope we can get these funds back.”
Notably, he revealed that pushing to «roll back» the Ethereum blockchain, which was suggested by some industry players on social media, including BitMEX co-founder Arthur Hayes, had been on the table for some time if the community agreed with it.
“I had my team talking to Vitalik and the Ethereum Foundation to see if there’s any recommendations they can offer to help. I do really thank all these guys on Twitter asking if there is a possibility to roll back the chain. I’m not sure what was the response on their side, but anything that would help we would try,” Zhou said.
When asked if «rolling back» the chain is even possible, Zhou responded he doesn’t know. “I’m not sure it’s a one-man decision based on the spirit of blockchain. It should be a work in process to see what the community wants,” he said.
It’s worth noting that a blockchain «rollback» refers to a state change that would allow for the funds to be recovered. While rolling back the Bitcoin blockchain is technically possible, such a state change on Ethereum would be more complex, given its smart contract interactions and state-based architecture.
Nevertheless, any state change would require consensus and likely lead to a contentious hard fork, drawing criticism from the community. This would likely split the Ethereum blockchain into two networks, each with its own supporters.
As for what exactly caused the hack to occur, is still unclear. Per Zhou, Bybit’s laptops have not been compromised. He said the movements of the transaction’s signers have been scrutinized but appear to have been routine.
“We know the cause is definitely around the Safe cold wallet. Whether it’s a problem with our laptops or on Safe’s side, we don’t know.,” Zhou added.
Uncategorized
Binance Research Survey Shows 95% of Latin American Crypto Users Plan to Buy More in 2025
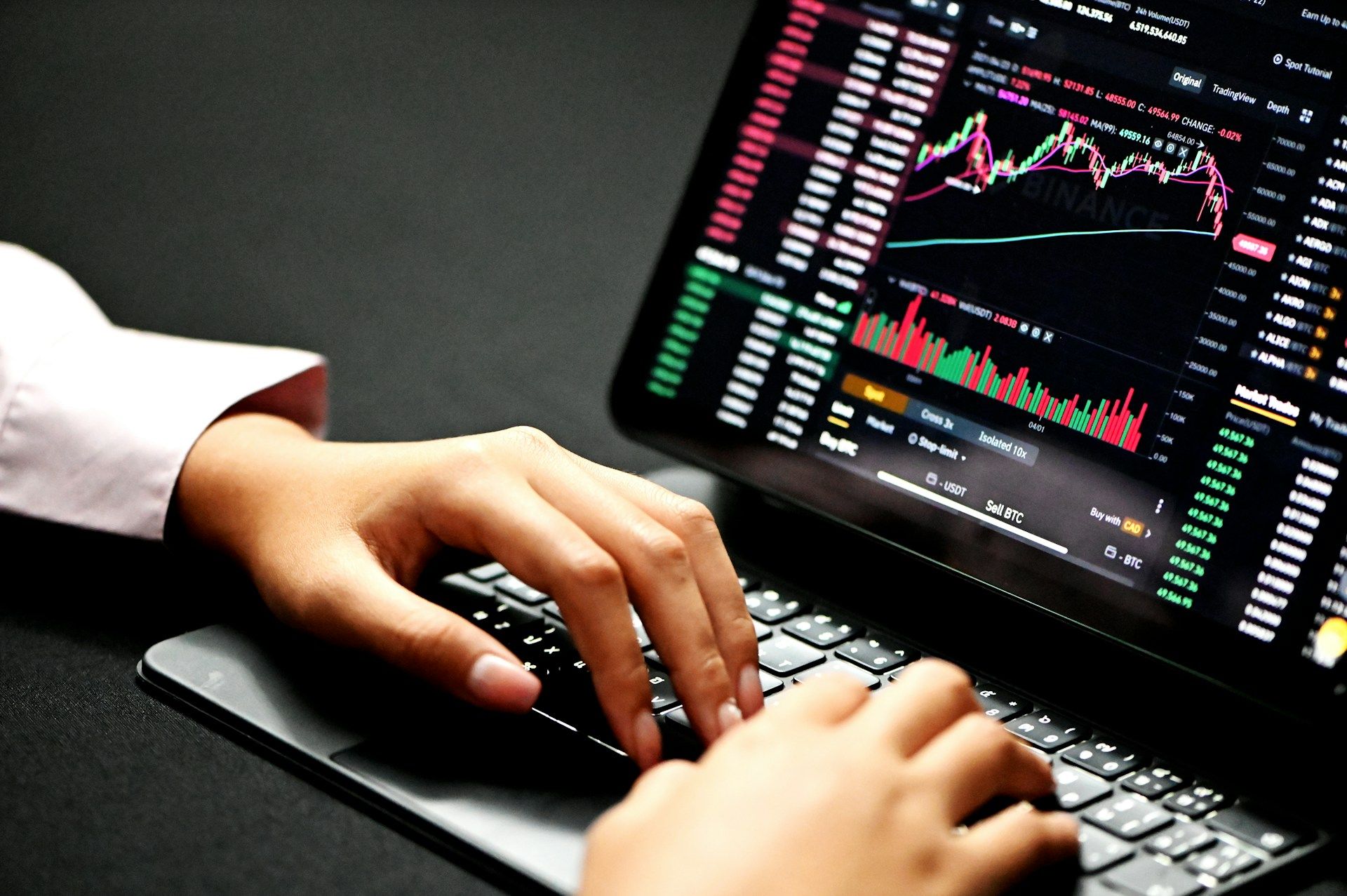
A vast majority of Latin American cryptocurrency users—95%—plan to expand their holdings in 2025, according to a Binance Research survey of more than 10,000 investors in Argentina, Brazil, Colombia, and Mexico.
The findings show that 40.1% of respondents are expecting to buy more crypto within the next three months, 15.3% are looking to do so in the next six months, and 39.7% within 12 months. Only 4.9% have no plans to keep on investing this year.
Latin America led the world in crypto adoption in 2024, growing by 116%, according to research from payments firm Triple-A quoted in the report. The region now has 55 million cryptocurrency users, making up nearly 10% of total cryptocurrency users.
This rapid expansion has been fueled by rising asset prices, regulatory advancements, and new financial products like spot bitcoin exchange-traded funds (ETFs). Brazil has just last week become the first country to approve a spot XRP ETF.
Market performance has also bolstered investor confidence. «Latin America is a rapidly expanding region for the crypto sector, and the results of this research reinforce what we have observed in our operations,” Binance’s regional VP for Latin America, Guilherme Nazar, said.
Binance’s research shows that half of those inquired already use cryptocurrencies for over a year, with most entering the space expecting significant returns and searching for financial freedom.
Portfolio diversification, privacy, and protecting their money were also quoted as motives to invest in the space.
Read more: How a $115M Crypto Fund With Big Ambitions Plans to Invest In Latin America
-
Fashion4 месяца ago
These \’90s fashion trends are making a comeback in 2017
-
Entertainment4 месяца ago
The final 6 \’Game of Thrones\’ episodes might feel like a full season
-
Fashion4 месяца ago
According to Dior Couture, this taboo fashion accessory is back
-
Entertainment4 месяца ago
The old and New Edition cast comes together to perform
-
Sports4 месяца ago
Phillies\’ Aaron Altherr makes mind-boggling barehanded play
-
Entertainment4 месяца ago
Disney\’s live-action Aladdin finally finds its stars
-
Business4 месяца ago
Uber and Lyft are finally available in all of New York State
-
Sports4 месяца ago
Steph Curry finally got the contract he deserves from the Warriors